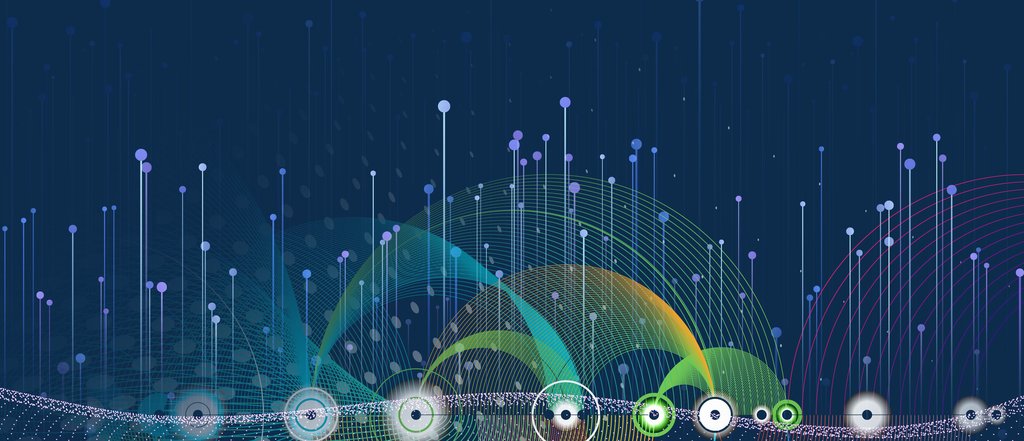
Seminars
Focused one-hour talks on the intersection of physical science and data science.
If you or your colleagues have used data science to advance physical science understanding and would like a chance to speak to the broader SUDS community, we’re eager to hear from you. Email us your information and the title and topic of your talk to start the conversation.
Discovering the Drivers of Global Air Quality Using Explainable Machine Learning
Providing accurate global estimates of air pollution is essential to evaluate the global public health burden of diseases associated with air pollution exposure. Nevertheless, their current knowledge of air pollution suffers from large biases in model predictions and insufficient information from the current observing system. The central objective of this SUDS effort is to provide new scientific insights into (1) the factors that control bias in air quality assessment, and (2) the drivers of global ozone trends and their impact on global air quality at scales relevant for assessing human health impacts. To address the objectives, the speakers have developed and utilize an explainable machine learning (ML) model to break down regional bias dependence and provide scientific interpretation of ozone and its bias drivers by analyzing a large set of exogenous and input data sources provided by JPL’s chemical data assimilation and various observations. They extracted and combined local and global measures of how inputs affected the physical model bias, providing ML model explanations and quantification of each parameter’s contribution. This effort will demonstrate a generalized approach for using explainable ML to identify, correct, and gain insight from primary drivers of physical model biases and variability while considering uncertainty.
Mapping Martian Frost with Machine Learning
The seasonal formation of martian surface frost provides the current best, global, multi-scale observational dataset for tracking the present-day volatile cycle on Mars. However, past Mars frost cycle studies have been limited by complete reliance on humans to manually parse and integrate large amounts of data from multiple, disparate observational records with scales varying from kilometers to meters. By bringing together physical and data scientists, we are creating a more systematic, unified approach combining visible, thermal, and spectral observations to generate a first-of-kind global frost formation map. Our initial steps include training a machine learning classification model to detect key frost-relevant surface features in visible imagery. Generating statistically robust training and evaluation datasets was critical and required a highly iterative annotation process, made more efficient with the use of Labelbox. In this presentation, we will outline the nuances of the frost detection practices of planetary scientists, how they were captured through iterative discussion and comparison with our human-generated labels, and finally how we evaluated and then improved the efficacy of our training set. Our lessons learned will apply directly to many image-based machine learning detection problems in physical science that must stand up to the rigor of later inference. This work is supported under the SUDS strategic initiative, which aims to create a community of practice involving collaborations between physical and data scientists.
Machine Learning for Global Detection of Fresh Impacts on Mars
Machine learning provides the ability to quickly sift through large data sets to identify rare observations of known scientific interest. This presentation will describe a collaboration between computer scientists and planetary scientists to use a machine learning classifier to search a global collection of Mars orbital observations for small, fresh impact craters. To date, a manual review of the most-confident machine learning detections has yielded 69 new discoveries of previously unknown impacts. The classifier has also helped reduce an observed bias in known impacts, which tend to be discovered in bright, dusty areas, by identifying candidates in darker, rocky areas as well. This presentation will describe the process of training and deploying the classifier as well as our review of the candidates it identified. Overall, the machine learning classifier serves to accelerate the process of scientific discovery by efficiently directing human attention to where it is most needed.
The Very Model of a Modern Time Domain Survey
Advances in instrumentation and data infrastructures are enabling a new generation of sky surveys which aim to repeatedly cover the visible sky on nightly to monthly cadences. These support a breadth of real-time scientific investigation from hunting for potentially hazardous asteroids to looking for the most distant cosmic explosions. They also generate unprecedented archives of billions of astronomical time series, enabling systematic studies of rare classes of star and galaxy. However, the data rates, volumes, and complexity are such that machine learning is necessary at all stages of the scientific process. In this talk, I will review the Zwicky Transient Facility, currently the largest public sky survey, and how data science is an essential component of its processing, analysis, and discovery pipelines.
Dying Glaciers, Rising Oceans, … and the Beauty of Gaussian Processes Regression
This talk provides an in-depth exploration of a single science question, “How much have glaciers contributed to sea level rise over the past 60 years?”, for which data science techniques are applied to help bring together disparate observations and modeling to advance our understanding of the Earth System. The talk takes a utilitarian perspective to advancing scientific understanding through embracing of data science.
Overview of Data Science for Physical Scientists
Physical scientists are already confronted with datasets that are too large (number of observations) and too complex (number of simultaneous parameters and context needed for understanding) to be fully interpreted by traditional analysis methods. Upcoming missions will transcend even these boundaries by orders of magnitude. Meanwhile, our physical models have become so complex and computationally intensive that translating model error into model improvement has become challenging. Data science, defined here as the techniques and approaches offered by machine learning, uncertainty quantification, and model-based inference, offer alternative paths to insight generation and rigorous conclusions that complement current analysis practices. This talk provides an overview of data science-enabled capabilities important for physical science analysis in the era of big, complex data. The goal of these capabilities are distinct from automation, autonomy, or industrial/commercial applications in that they focus on facilitating insight and understanding. This seminar and the series that follows seeks to stimulate new conversations and collaborations between JPL's physical and data scientists and a growing familiarity with each other’s vocabulary, needs, and state-of-the-art efforts.
CL#23-1518