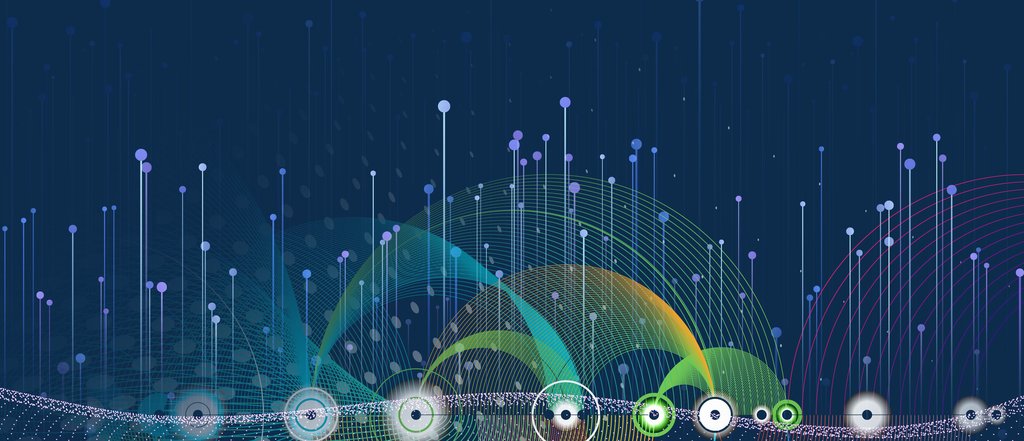
SUDS Report
The SUDS initiative is guided by the findings and recommendations captured in the report produced during our initial council activity. The full report is available as a PDF through the link below.
Executive Summary
The Science Understanding from Data Science (SUDS) Council prime mission, as defined in the commissioning charter, was to develop a roadmap to advance JPL leadership, in collaboration with Campus, in scientific understanding based on state-of-the-art data science methodologies. This report represents the results of that strategic vision exercise that was grounded on informed analysis of the current state of practice within JPL and in the external community. Our Council membership included diverse representation across the different science and engineering organizations, as well as Caltech, to provide multiple points of view on how JPL science research is currently working with JPL and Caltech data scientists and how data science is supporting scientific research. Council members were expected to “wear the big hat.” Data science in this activity is specifically defined to include model-based inference, uncertainty quantification, and machine learning. Central to the SUDS Council vision is the focus on direct scientific research application; application to other areas such as engineering telemetry, onboard autonomy, intelligent instruments, and other areas potentially of high value to JPL, were outside the scope of the review and recommendations captured in this report.
In order to keep the analysis and recommendations science driven, we defined our success metrics as both the standard measure of scientific productivity—journal articles with many citations—as well as other measures of benefit to scientific research including increased speed and agility of our science by using fewer hours to achieve the same results, or more comprehensive results, that provide a deeper understanding of underlying scientific processes; and enhanced rigor and reproducibility.
In reviewing the current state of the science practice of applying data science to science we found that Astrophysics, Earth Science, and Planetary science have distinctly different degrees of infusion of data science techniques within their fields. Astrophysics at JPL works intensely in machine learning and model-based inference. Earth Science leads in mature model-based inference and uncertainty quantification. Planetary Science was just beginning to engage with machine learning and model-based inference. Differences between science areas are related to the volume of available data, simultaneous overlapping instrument coverage, the existence and maturity and complexity of physical models, and the balance between exploration, that is making new science discoveries, and characterization, i.e., understanding an underlying process. The Astronomy and Earth science missions have generated massive data sets, with a need to constrain confounding factors in turning photons into observations. Compared to the other two fields, Earth science has extensive independent data sets for validation of uncertainty quantification as well as a great need to understand our confidence in results before being used for societally relevant applications. Planetary science is starting to see challenges associated with large data sets that other fields have experienced. It is also in the unique position of sitting behind a massive downlink barrier, transforming much of its data science support into onboard science instrument autonomy to recognize and capture events while minimizing and characterizing induced sampling biases.
For the broader JPL landscape of how well data scientists are working with scientists, we found that there are islands of very successful collaborations, but there were challenges to these projects radiating their expertise and success out to the broader community. Given that the Council sees a growing need for better data science techniques and expertise for research, our recommendations are focused on addressing the current obstacles to knowledge diffusion, scaling up the successful collaborative formula, and developing a coherent community of practice. We found four data science capabilities that have been most useful for scientific understanding: model acceleration, explorations, insight generation, and rigor.
The council identified the obstacles to fully reaching our potential in applying data science for science as falling in six broader categories. First, there are cultural, communication, and perceptual challenges—both of data science within the research community and of scientific research from data scientists—that need to be overcome. Second, there is a need to establish standards and fair for collaborators from both domains. Third, there is a need for targeted, accessible cross-training in science and data science to grow multidisciplinary careers. Fourth, there are infrastructure challenges where current JPL resources are not suited to the needs of the research-level science project activities that are often much smaller in budget and resource needs than missions but are necessary incubators for our future mission science. Fifth, there are mismatches in the NASA funding structure, the main lifeline for scientific research, that can place unnecessary challenges on science and data science collaborations. The sixth and final obstacle is a fragmented organizational structure and missing role definitions to incentivize and grow scientific data science applications.
The proposed recommendations and roadmap are designed to address the above primary challenges. Prior to this council’s work, it was hypothesized that the formation of a community of practice would be desirable to nurture and connect specialized data science skills with relevant science users and use-cases. The council’s highest-level finding identified that achieving a vibrant, highly collaborative community will require the creation of a shared culture that transcends the current organizational and cultural differences between JPL scientists and data scientists. Further, there was strong desire from both the scientists and data scientists for this deep, collaborative relationship. The cultural principles of this community would include an emphasis on the top-level shared responsibility between physical scientists and data scientists to achieve impactful science output, acknowledgement that each member is both a mentor and ambassador in an evolving community, and a mutual respect for the setting and achieving of an appropriate level of rigor and defensible results for the science question at hand. This community of practice would include Caltech and university partners, recognizing that inclusion of academic researchers will bring in complementary expertise as well as students and postdocs who can sustain the cultural principals and community of practice going forward.
To provide an overarching structure for our detailed recommendations, we envision establishing a virtual group co-led by a physical scientist and data scientist with sufficient autonomy to nurture deep, mutual collaboration centered on selected science use-cases. Successful execution of the strategy will measurably improve JPL’s scientific understanding and productivity within Astrophysics, Earth, and Planetary sciences by infusing data science techniques for direct science support such as uncertainty quantification, model-based inference, machine learning, and artificial intelligence.
Understanding that a community is built on more than colocation, our recommendations consist of several elements. These elements include training and intuition-building activities, establishing standards for software and tools to improve ease of sharing and reuse, infrastructure improvements, defining mutual expectations for productive collaborations, future organizational and role definition concepts, and several high science impact trail-blazing projects to provide a common purpose and the explicit mandate to capture their collaborative process for later sharing and replication.
CL#23-1518